
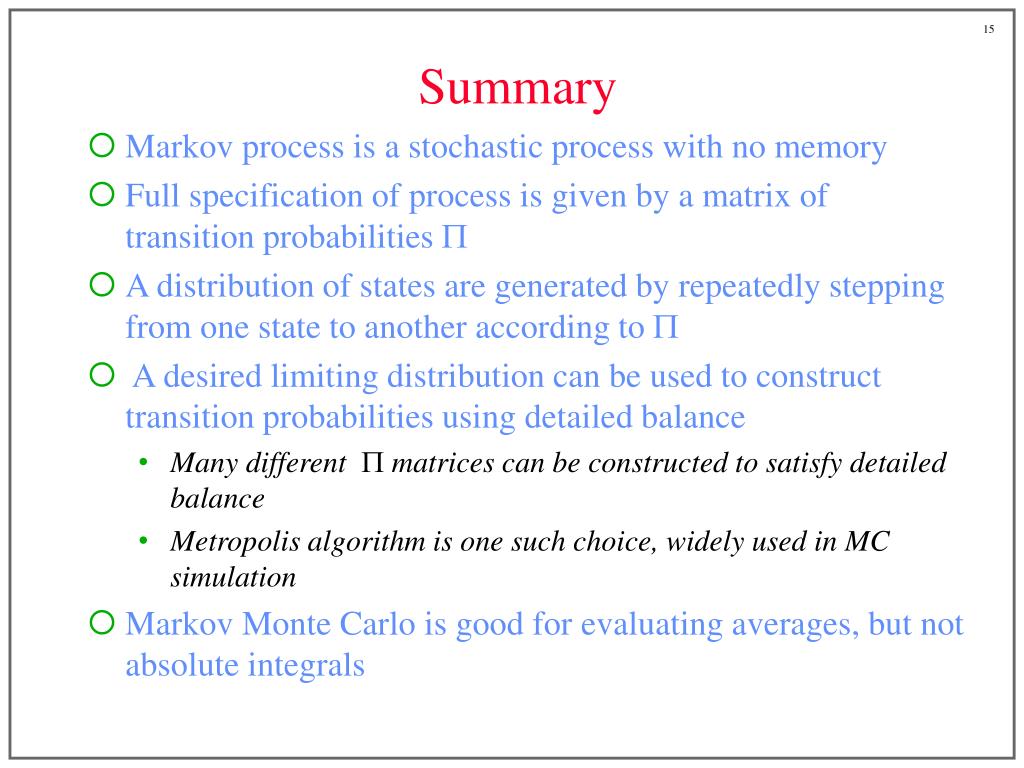
Markov chains are powerful for problems modelling when dealing with random dynamics. We can use a discrete-time Markov chain to describe such movement using the transition probabilities between the health states. If we classify the chronic disease into distinct health states, the movement through these health states over time then represents a patient’s disease history. The main idea is that everyone is Healthy, then gets the disease and finally dies.Ī discrete-time Markov chain with stationary transition probabilities is often used to investigate treatment programs and health care protocols for chronic disease. A more complete description of these models can be found in Mathematical Epidemiology by Brauer.Ĭhronic disease models do not usually have interaction between compartments, think in diabetes which is not contagious. For example, you can divide the infected into symptomatic and asymptomatic, you can divide the susceptible into vaccinated and unvaccinated, removed can be divided into recovered or death. Also, there are a lot of infectious disease models that use this idea and add additional compartments. The interesting part is how interactions between the compartments give rise to different models. The main idea is that almost all individuals start Susceptible they can catch the disease with a handful of Infected.Ĭonsidering the discrete-time, such as days, some infected individuals will either get better or die, hence becoming Removed, and some Susceptible will become Infected.
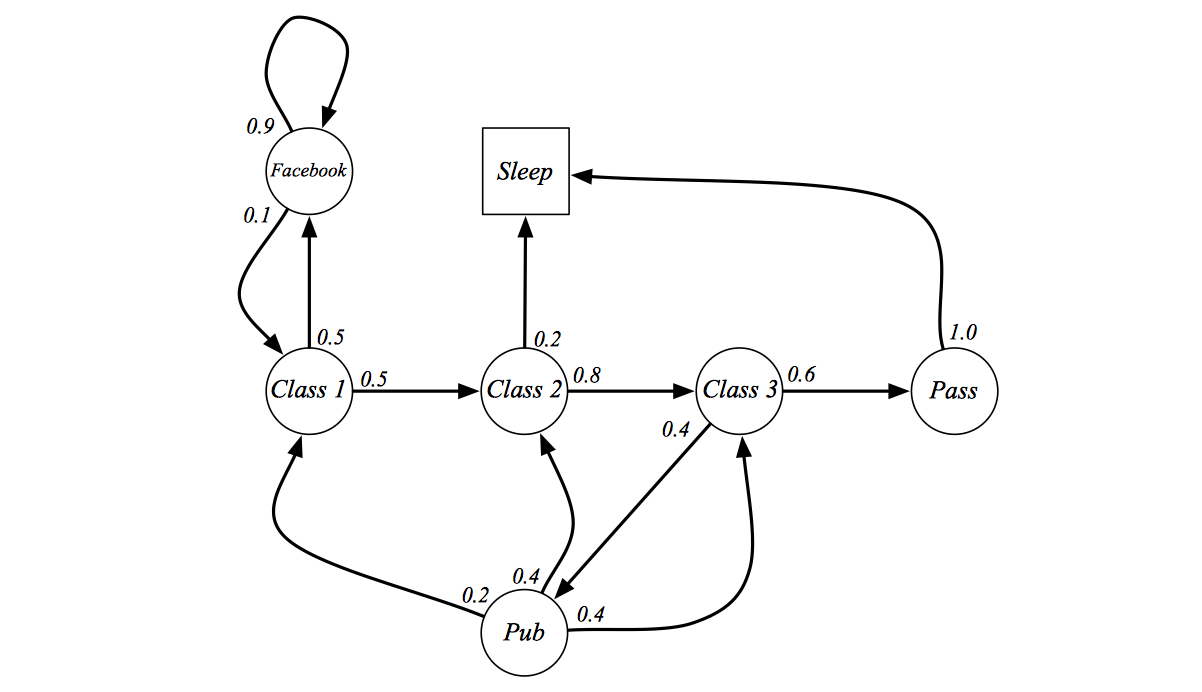
The main idea is that individuals can be classified into three states: Susceptible (people that can get the disease), Infected (people that have the disease and are contagious), and Removed. The most famous is the SIR model for infectious diseases. The Markov Chains are usually called in the epidemiological and in the chemistry literature “compartmental models”. Other areas of application include predicting asset and option prices and calculating credit risks. In economics and finance, they are often used to predict macroeconomic situations like market crashes and cycles between recession and expansion. These fields range from the mapping of animal life populations to search engine algorithms, music composition and speech recognition. Since Markov chains can be designed to model many real-world processes, they are used in a wide variety of situations. However, an infinite-state Markov chain does not have to be a steady-state, but a steady-state Markov chain must be time-homogenous. This phenomenon is also called a steady-state Markov chain where the probabilities for different outcomes converge to a certain value. A continuous-time Markov chain changes at any time.Ī Markov chain can be stationary and therefore be independent of the initial state in the process. The value of the Markov chain in discrete-time is called the state and in this case, the state corresponds to the closing price. One example to explain the discrete-time Markov chain is the price of an asset where the value is registered only at the end of the day. In this article, we will focus on discrete-time Markov chains. This means that we have one case where the changes happen at specific states and one where the changes are continuous. This is certainly valid if the state space is finite, which is the only case we analyze in what follows.When approaching Markov chains there are two different types: discrete-time Markov chains and continuous-time Markov chains. We have assumed that the sum and the limit in (6.1.3) can be interchanged.Simultaneous linear differential equations appear in so many applications that we leave the further exploration of these forward and backward equations as simple differential equation topics rather than topics that have special properties for Markov processes.
